Measuring the Poverty in Myanmar: A View of Health Expenditure
Khin Sandi Myint
School of Statistics, Jiangxi University of Finance and Economics, Nanchang, Jiangxi 330013, China
Department of Statistics, Nay Pyi Taw State Academy, Nay Pyi Taw, Myanmar
ksdm20619@gmail.com
Abstract
This paper demonstrates the utilization of a quantile regression model to empirically analyze Myanmar’s poverty situation concerning three key factors: current health expenditure, gross domestic product (GDP), and total unemployment. The primary objectives are to assess the poverty level using health indicators, income indicators (GDP), and total unemployment and to investigate the significance factor of poverty. The study also explores the specific percentiles or quantiles at which unemployment and GDP impact the current health expenditure indicator. The findings reveal that gross domestic product significantly impacts health expenditure across all quantile levels. It is noteworthy that the conditional median in LN (health expenditure) is due to LN (unemployment) and LN (GDP). Thus, quantile regression provides a more comprehensive understanding of the conditional distribution of the dependent variable. Poverty can be directly affected by gross domestic product and unemployment. The government should create job opportunities and economic growth and employ comprehensive policies to enhance living conditions and break the cycle of poverty, illiteracy, and unemployment.
Keywords: Poverty, Gross Domestic Product, Unemployment, Current Health Expenditure, Least Square Regression, Quantile Regression
I. Introduction
Nowadays, poverty remains a significant global issue, exacerbated by the aftermath of the COVID-19 pandemic and its adverse economic consequences. Measuring poverty varies depending on the perspective and emphasized aspects, leading to diverse approaches. Poverty represents a condition where individuals lack the essential material resources and income to meet their basic needs. Poverty takes two primary forms: absolute and relative. Absolute poverty entails severe deprivation of fundamental human necessities. Its causes include debt, population growth, conflicts, and natural disasters. Relative poverty is defined by household income falling below a set percentage of the median income, limiting full participation in society and everyday opportunities.
Various indicators measure poverty, with the most commonly utilized ones being HDI (Human Development Index), expenditure approach (GDP), the income approach (GDP), poverty index, literacy rate, migration, employment, and inflation. This study uses least square (LS) and quantile regression to explore the impact factors on expenditure of health by gross domestic product. The data spans between 1991 and 2022 and is sourced from the World Bank, internet websites, and the ministries in Myanmar. Moreover, it emphasizes the applications of LS regression and quantile regression and the ability to improve interpretation and enhance inference of quantile regression.
Many prior studies have delved into the examination of poverty through the lenses of various indicators such as gross domestic product (GDP), Human Development Index (HDI), employment rates, income levels, and consumption inequality. Iradian (2005) investigation specifically focused on income inequality and its correlation with economic growth as a measure of poverty. This current research aims to enhance the understanding of poverty dynamics in Myanmar by incorporating an analysis of current health expenditure relative to GDP and total unemployment. The identification of research objectives involves a thorough exploration of the impact of unemployment conditions on current health expenditure in conjunction with gross domestic product.
This study employs comparison analysis between the LS regression and quantile regression. LS regression is a statistical method used for modeling the relationship between a dependent variable and one or more independent variables by minimizing the sum of the squares of the differences between observed and predicted values. The goal is to find the best-fitting line (or curve in the case of multiple independent variables) that minimizes the sum of squared residuals, which are the differences between the observed values and the values predicted by the model. There are some assumptions of LS regression. (1) The relationship between the independent variable(s) and the dependent variable is assumed to be linear. (2) The residuals should be independent of each other. (3) The variance of the residuals should remain constant across all levels of the independent variable(s), or there is homoscedasticity. (4) The residuals are assumed to be normally distributed. (5) There should be no perfect linear relationship among the independent variables or no perfect multi-collinearity (Gujarati, 2013).
Chambers (1996) mentions that quantile regression is utilized to investigate the impact of one or more independent variables on various quantiles (percentiles) of the dependent variable. It extends beyond linear regression and proves valuable when linear regression assumptions, such as linearity, homoscedasticity, and normality, are not satisfied. Furthermore, quantile regression remains applicable even in the absence of heteroscedasticity within the data. It has no strong distributional assumptions, but it assumes a linear relationship between predictor variables and the conditional quantiles of the response variable, along with the assumption that observations are independent. To ensure stability in parameter estimates, avoiding multi-collinearity, where predictor variables exhibit high correlation, is crucial. Unlike linear regression, quantile regression does not assume a constant variance of residuals or the normal distribution of errors. Typically, quantile regression finds its most common application in estimating the response variable’s median (50th percentile). Thus, the mean can serve as a test statistic in regression analysis, whereas in quantile regression, the test statistic employed is the median (Das et al., 2019). This study outlines a detailed, step-by-step procedure for conducting quantile regression analysis.
II. Literature Review
In Myanmar, the poverty rate in rural areas is 1.8 times higher than that in urban areas (UNDP, 2013). In stark contrast to regions such as Yangon, Mandalay, and Tanintharyi, the poverty rate in Chin region is found to be four times higher. Similarly, Rakhine exhibited a poverty rate that was three times greater than the aforementioned regions. These disparities underscore the significant economic challenges faced by the population in Chin and Rakhine, emphasizing the pressing need for targeted interventions and socio-economic development initiatives in these areas. The pronounced contrast in poverty rates highlights the multifaceted nature of economic disparities across different regions within Myanmar, necessitating a nuanced approach to poverty alleviation strategies that considers the unique challenges faced by each locality (UNDP, 2019). Furthermore, Myanmar successfully narrowed the poverty gap across all states and territories from 2005 to 2010.
Myanmar has made commendable strides in recent years, successfully reducing household-level poverty and achieving notable progress on the socio-economic front. Despite these commendable advancements, a sobering reality persists: nearly one-third of children in Myanmar continue to reside in disadvantaged households. This statistic underscores the ongoing challenges and disparities that persist, particularly impacting the well-being and prospects of the younger generation. The need for targeted interventions and sustained efforts to address the root causes of child poverty remains imperative, ensuring that the positive momentum in poverty reduction is extended to encompass vulnerable segments of the population, thereby fostering comprehensive and inclusive socio-economic development. The juxtaposition of overall poverty reduction with the persisting challenges faced by a significant portion of Myanmar’s youth serves as a poignant reminder of the nuanced nature of poverty alleviation efforts and the importance of addressing disparities at all levels of society (UNICEF, 2022). Moreover, child poverty rates outpaced those of adults, primarily due to the higher occurrence of larger families in financially disadvantaged households. Child poverty was more pronounced in rural areas than in urban environments and disproportionately affected younger children in comparison to their older counterparts.
In Myanmar, the roots of poverty were multifaceted, encompassing factors such as a less robust foundation of productive and financial assets, coupled with limited access to essential social services. To chart a path towards sustainable development, a strategic second phase of reforms was initiated, with a primary focus on financial reforms and inclusion. This transformative phase was marked by heightened public investment in critical areas such as infrastructure, education, and healthcare. The bolstering of these sectors was made possible through improved revenue generation and augmented by external funding sources. These comprehensive efforts were strategically aligned with the objective of advancing Myanmar’s progress toward attaining the Sustainable Development Goals, reflecting a commitment to addressing the intricate web of challenges contributing to poverty in the country (Dynamics, 2017).
Myanmar’s National Strategy for Rural Development and Poverty Reduction strategically identified eight pivotal sectors, including health, education, and social protection, with increased financial support. Additionally, continuous efforts were underway to enhance the capacities of local governance through several initiatives: (i) establishing planning and implementation committees at the national, provincial, and local levels; (ii) fostering community-driven development organizations and decentralized management of social services; (iii) formulating inclusive, township-driven development plans. These measures aimed not only to strengthen the foundational pillars of rural development but also to empower local communities through participatory governance and community-led initiatives, marking a holistic approach towards sustainable poverty reduction in Myanmar (Asian Development Bank, 2019).
The World Bank’s poverty line estimate for developing countries was established at $2 daily per capita, whereas in South Africa, the household poverty threshold was determined to be R800 per month, utilizing 1996 price standards (Hare, 2002). In India, 7% of the populace experienced extreme poverty, with 0.6 Indians lifting themselves out of such conditions every minute. The significant allocation of funds by the Indian government to rural welfare initiatives played a crucial role in alleviating national poverty. The government aimed to eliminate extreme poverty, defined as individuals living on less than $1.25 daily for all citizens by 2030. The economic decline linked to the COVID-19 pandemic was projected to have caused a temporary surge in both poverty and inequality in India (Arbatli Saxegaard, 2023). Moreover, this study investigated the dynamics of income and consumption among households across various income percentiles. According to the World Bank’s assessment using the lower-middle-income poverty rate in Pakistan, the poverty ratio was calculated at 39.3 percent in 2020-21. Projections suggested that it was expected to persist at 39.2 percent in 2021-22 and potentially decrease to 37.9 percent by 2022-23.
Economic growth emerged as a powerful tool for alleviating poverty and improving the quality of life in developing nations. Robust evidence from thorough cross-country investigations and individual country analyses strongly underscored that rapid and sustained growth was crucial for expediting progress toward the realization of the Millennium Development Goals (Rodrik, 2007). Approximately 4 million individuals were unable to adhere to modern nutritional guidelines due to insufficient financial resources, preventing them from accessing fresh fruits, vegetables, or even securing two meals a day (Pantazis et al., 2006). Moreover, in excess of 10.5 million individuals wrestled with financial instability, which hindered their ability to save, protect their household possessions, or partake in even the most modest personal indulgences.
Al-Zaman (2023) delved into the intricate relationship between information poverty and a diverse array of topics discussed within the publication. These encompass social justice, rural areas, information rights, access to information, social exclusion, information management, information processing, socioeconomic factors, poverty, and health services, all intricately interwoven with the overarching concept of information poverty. A clear implication arose from the idea that shifting the growth pattern towards sectors with robust employment opportunities was likely to yield the most substantial and direct impact on poverty reduction. By strategically channeling economic development into sectors that not only foster job creation but also promote sustainable and inclusive growth, the potential for alleviating poverty becomes more pronounced. This strategic approach not only addresses the immediate need for increased employment but also sets the stage for long-term socioeconomic advancement, creating a more resilient and equitable foundation for poverty reduction efforts (Weiss, 2005).
Biyase & Zwane (2018) conducted an insightful analysis, discovering that both fixed-effect and random-effect analyses unveiled the significance of several factors in influencing household welfare. Notably, the education level of the household head, specific province dummies, the household head’s race, dependency ratio, gender, employment status, and marital status emerged as statistically significant determinants. These findings underscore the complex interplay of various socio-economic factors in shaping the well-being of households. In response to these insights, ongoing efforts have prioritized sustained investments in education and targeted improvements in the economic conditions of rural residents in traditional rural areas. Recognizing the pivotal role of education and economic empowerment, these endeavors represent a focused strategy in the broader framework of South Africa’s poverty alleviation initiatives. The commitment to addressing these influential factors is integral to creating lasting and meaningful improvements in household welfare across diverse communities in the country.
Iradian (2005) delved into the intricate connections between economic growth and income inequality, meticulously examining the impact of growth, inequality, and government expenditure on poverty alleviation. The findings of the research brought to light the nuanced dynamics at play in the relationship between these variables. Interestingly, the results indicated that income inequality had a discernible and adverse effect on economic growth, aligning with the established principles of the Kuznets curve. In the short term, a positive association between income inequality and growth was discerned, suggesting a complex interplay between these factors during certain phases of economic development. However, over the long term, this relationship underwent a shift, turning negative and revealing the potential challenges posed by persistent income inequality to sustained economic growth. These insightful revelations contribute valuable nuances to our understanding of the intricate dynamics between economic growth, income inequality, and their cumulative impact on poverty alleviation. Recognizing the temporal dimensions of these relationships is crucial for formulating targeted and effective policies geared toward fostering sustainable economic development and mitigating the adverse effects of income inequality on long-term growth.
The prevalence of extreme poverty in Sub-Saharan Africa (SSA) was increasing, and projections indicated that by 2030, it would exceed the total population of the European Union (Flento, 2021). Moreover, The United Nations, in collaboration with the World Bank, endeavored to universalize the concept of poverty by establishing a common definition known as absolute poverty. This definition was operationalized into a poverty threshold of US$1.90 per day with the assistance of the World Bank. Rural poverty in China is characterized by an annual per capita income of less than 2,300 yuan (approximately US$350) (ILO, 2020).
The primary objective of classical regression, as elucidated by (Chambers, 1996), was to estimate the conditional means of a response variable through the examination of the values of the explanatory variables. However, the efficacy of classical regression hinged heavily on the fulfillment of regression assumptions, and its reliability tended to diminish in nonstandard conditions. Recognizing the limitations in such scenarios, an alternative and robust approach emerged in the form of quantile regression, as advocated by Das et al. (2019). Quantile regression proved to be a valuable technique for data analysis, especially when traditional regression assumptions were not met. This method offered a more versatile and resilient tool for exploring relationships in datasets characterized by heterogeneity or deviations from standard assumptions, thereby enhancing the analytical toolkit available for researchers and practitioners alike.
III. Methods and Material
Least Squares Regression is a statistical method used to model the relationship between a dependent variable and one or more independent variables by minimizing the sum of the squared differences between the observed and predicted values (Jaeger, 2006). LS regression model is
Y=β_0+β_1*X_1+ β_2*X_2+ε_i
Y is the dependent variable.
X_i is independent variables.
β_0 is the intercept.
β_1 is the slope.
ε_i is the random error term.
Quantile regression is a statistical technique used to model the relationship between a dependent variable and one or more independent variables while focusing on specific quantiles of the dependent variable’s distribution (Das et al., 2019). The quantile regression model is
Y(τ)=β_0 (τ)+β_1 (τ)*X_1+β_2 (τ)*X_2 +ε_i
Y(τ) is the〖 τ〗^(th )quantile of the dependent variable
β_0 (τ) is the intercept of the 〖 τ〗^(th )quantile
β_1 (τ) is the coefficient for the independent variable X_1 for the 〖 τ〗^(th ) quantile
β_2 (τ) is the coefficient for the independent variable X_2 for the 〖 τ〗^(th ) quantile
ε_i is the random error term.
IV. Results
In this study, the dependent variable is current health expenditure per capita and independent variables are GDP per capita (LCU), and total unemployment (%of total labor force). The hypothesis is that to explore unemployment and GDP per capita are statistically significance on current health expenditure per capita or not and to assess poverty levels using health indicators, income indicators (GDP), and total unemployment and to investigate the significance factor of poverty. Thus,
H_0: There is no statistically significance between dependent variable and independent variables.
H_1: There is statistically significance between dependent variable and independent variables.
The estimated equation is
Current health expenditure per capita = c (1) *unemployment (% of total labor force) + c (2) *GDP per capita + c (3)
Initially, during the assessment of certain assumptions, this study reveals deviations from the normality assumption, along with the presence of both serial correlation and heteroscedasticity in the data. Consequently, the utilization of quantile regression becomes warranted for the analysis.
Based on Jarque -Bera test, the residuals do not follow the normal distribution since the P- value is 0.016594 and it is less than 0.05. Then checking the serial correlation and heteroscedasticity, the results in table (1) show that there are both serial correlation and heteroscedasticity as well as the residuals do not follow normal distribution. Thus, the findings indicate that the data deviates from the assumptions inherent in least squares regression. Therefore, employing quantile regression emerges as a suitable approach for analyzing the dataset.
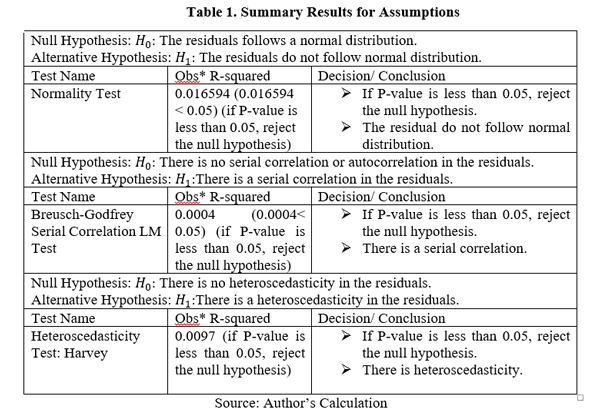
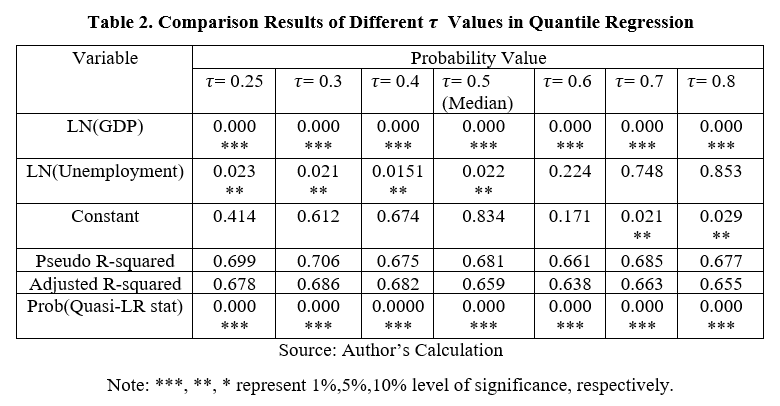
Table (2) also shows the Pseudo R-Squared and Adjusted R-square for corresponding τ values. This study finds that 66% variation in the conditional median in current health expenditure is due to LN (GDP per capita) and LN (Unemployment). P-values (Quasi-LR stat) are less than 0.05 which indicate that the model is stable for all quantile levels.
Table (3) illustrates LN (GDP per capita) and LN (Unemployment) are significance impact on current health expenditure per capita at 5% level of significance by using the least squares regression. In table (4) finds that conditional median in LN (health expenditure) is due to LN (unemployment) and LN (GDP per capita) by using the quantile regression. Moreover, quantile regression enables the precise determination of the significance percentile or quantile. This precise significant quantile finds in Table (5).
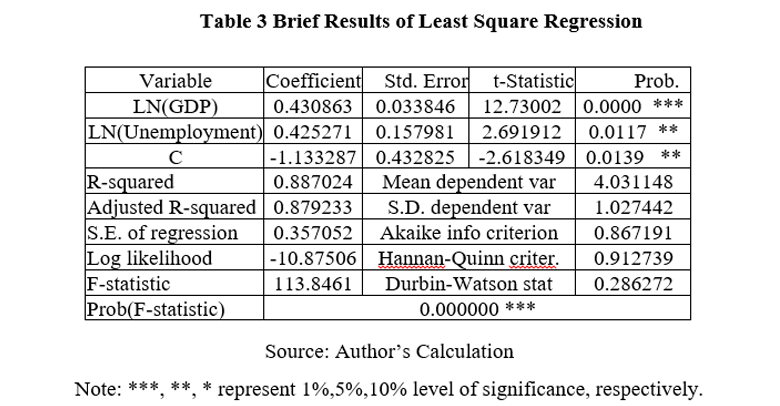
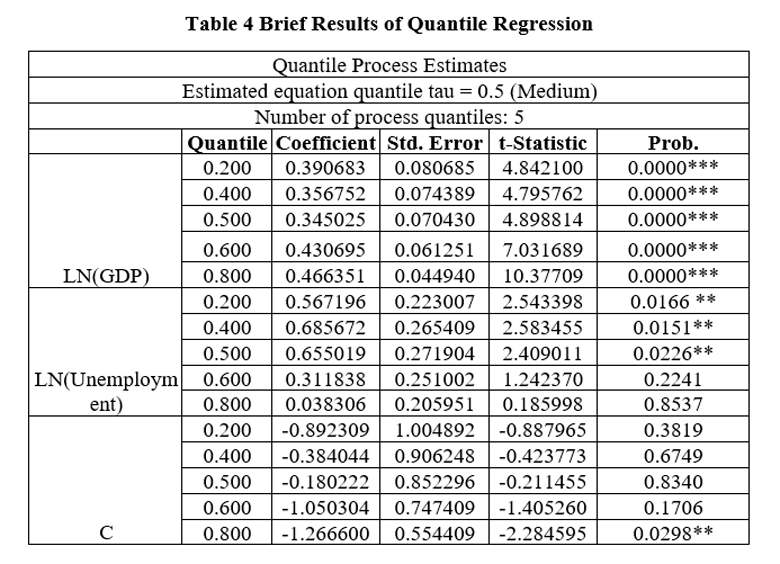
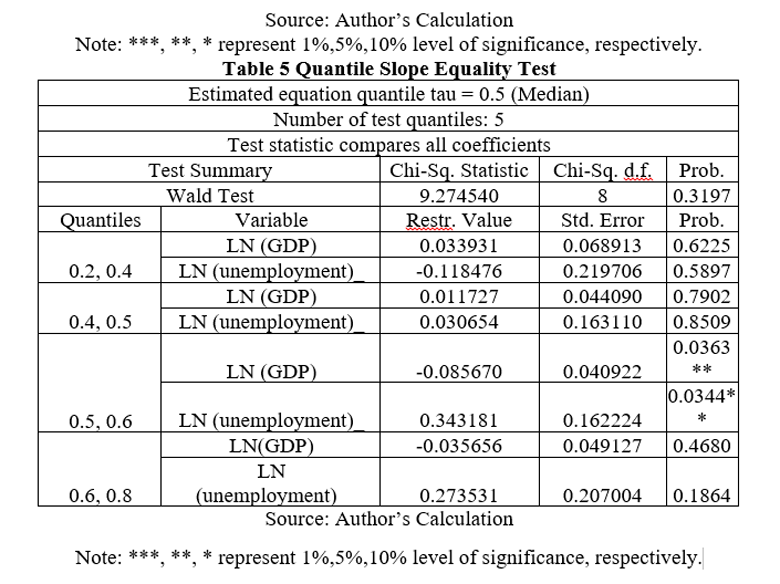
V. Conclusion and Discussions
In summary, this study not only affirms the impact of gross domestic product (GDP) per capita and total unemployment on current health expenditures, as demonstrated through both least square and quantile regression methods, but also underscores the link between insufficient economic development, unemployment, and the prevalence of poverty. Addressing this complex issue necessitates proactive measures from governments, including the creation of ample employment opportunities. The formulation and promotion of policies geared toward stimulating economic growth through strategic investments in infrastructure, education, and technology are crucial steps in this direction. Furthermore, the study emphasizes the utility of both least square regression and quantile regression, with a particular spotlight on the latter. The findings underscore that quantile regression not only enhances the interpretability of results but also enriches the inference process, presenting several advantages over traditional least squares (OLS) regression. This insight positions quantile regression as a valuable and versatile technique, especially when dealing with datasets that deviate from the assumptions of linear regression. In a broader context, this paper contributes significantly to the evolving literature addressing the impact of health expenditure, unemployment, and economic growth. By providing valuable insights into policy responses to the interconnected challenges of poverty, unemployment, and economic development, the study contributes to the ongoing discourse on formulating effective strategies for fostering sustainable and inclusive socio-economic progress.
References
Al-Zaman, S. (2023). A review of literature on information poverty. GeoJournal, 88(5), 4907–4920. https://doi.org/10.1007/s10708-023-10901-x
Arbatli Saxegaard, E. (2023). Inequality and Poverty in India: Impact of COVID-19 Pandemic and Policy Response. IMF Working Papers, 2023(147), 1. https://doi.org/10.5089/9798400247873.001
Asian Development Bank. (2019). MYA : Resilient Community Development Project (Issue October).
Biyase, M., & Zwane, T. (2018). An Empirical Analysis of the Determinants of Poverty and Household
Welfare in South Africa. The Journal of Developing Areas, 52(1), 115–130. https://doi.org/10.1353/jda.2018.0008
Chambers, J. K. (1996). Quantitative Applications in the Social Sciences. In Journal of English
Linguistics (Vol. 24, Issue 2). https://doi.org/10.1177/007542429602400208
Das, K., Krzywinski, M., & Altman, N. (2019). Quantile regression. In Nature Methods (Vol. 16, Issue 6). https://doi.org/10.1038/s41592-019-0406-y
Dynamics, A. P. (2017). POVERTY DYNAMICS AND SUSTAINABLE DEVELOPMENT GOALS ( SDGS ) 1.
Flento, J. (2021). Development Economics. August.
Gujarati, D. & P. D. (2013). Basic Econometrics. In Introductory Econometrics: A Practical Approach.
Hare, L. B. (2002). Chapter one. In Quality Progress (Vol. 35, Issue 8, pp. 77–79). https://doi.org/10.7829/j.ctv1w0xcfk.4
ILO. (2020). Beyond the goal of eradicating absolute poverty in China: relative poverty indicators and social security policies. International Labour Organization, 1, 1–3.
Iradian, G. (2005). Inequality , Poverty , and Growth : Cross-Country Evidence.
Jaeger, B. (2006). Brown university – The method of least squares. Handbook of Research on Informatics in Healthcare and Biomedicine, 181–184.
Pantazis, C., Gordon, D., & Levitas, R. (2006). Poverty and social exclusion in Britain. Poverty and Social Exclusion in Britain. https://doi.org/10.56687/9781447366843
Rodrik, D. (2007). Growth Building Jobs and prosperity in Developing Countries. Why Growth Should Be at the Heart of Development Policy, 1–25.
UNDP. (2013). UNDP_MM_PvR_RegionalPovertyReport.pdf. http://www.mm.undp.org/content/dam/myanmar/docs/Publications/PovRedu/UNDP_MM_PvR_RegionalPovertyReport.pdf
UNDP. (2019). Conditions Survey 2017 (Issue June).
UNICEF. (2022). Overcoming Child Poverty in Myanmar. https://www.unicef.org/myanmar/media/5806/file/Overcoming Child Poverty in Myanmar.pdf
Weiss, J. (2005). Experiences with poverty targeting in Asia: An overview. Poverty Targeting in Asia, 1–33. https://doi.org/10.4337/9781845424701.00008
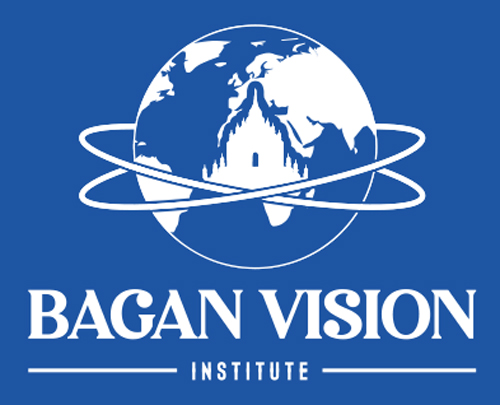
Address
E-304, Mya Kyi Ryar 2nd Street, Htan Ta Pin Special Block, Dekkina Thiri Township, Naypyitaw.
Phone
(+959) 945 404 777
(+959) 882 640 707